Fine-Tuning vs. Pre-Training: How to Choose for Your AI Application
Imagine you are standing in a grand library, where the books hold centuries of human thoughts. But you are tasked with a singular mission: find the one book that contains the precise knowledge you need. Do you dive deep and explore from scratch? Or do you pick a book that’s already been written, and tweak it, refining its wisdom to suit your needs?
This is the crossroads AI business and developers face when deciding between pre-training and fine-tuning. Both paths have their own fun and challenges. In this blog, we explore what lies at the heart of each approach: definitions, pros and cons, then the strategy to choose wisely.
Introduction
What is Pre-Training?
Pre-training refers to the process of training an AI model from scratch on a large dataset to learn general patterns and representations. Typically, this training happens over many iterations, requiring substantial computational resources, time, and data. The model, in essence, develops a deep understanding of the general features within the data, and can be used for inference at convenience.
The outcome of pre-training is usually a relatively stable, effective model adapted to the application scenarios as designed. It could be specified on a certain data domain and particular tasks, or applicable to general usage. Typical examples of pre-trained models include large language models like ChatGPT, Llama, Claude; or large vision models such as CLIP.
What is Fine-Tuning?
The fine-tuning process usually takes a pre-trained model and adjusts it to perform a specific task. This involves updating the weights of the pre-trained model using a smaller, task-specific dataset. Since the model already understands general patterns in the data (from pre-training), fine-tuning further improves it to specialize in your particular problem while reusing the knowledge from pre-training.
This process is often quicker and less resource-intensive than pre-training, as the pre-trained model already captures a wide range of useful features. And more importantly, the task-specific dataset is usually small. Fine-tuning is widely used especially for domain specialization, recent developments include finance, education, science, medicine, etc.
A Little More on the History
The liaison between fine-tuning and pre-training does not emerge in the era of LLMs. In fact, the development of deep learning brings about a perspective of these two “routines”. We can view the brief history of deep learning as three stages with respect to pre-training and fine-tuning.
- First stage (20thcentury): supervised fine-tuning only
- Second stage (early 21stcentury to 2020): supervised layer-wise pretraining + supervised fine-tuning
- Third stage (2020 to date): unsupervised layer-wise pretraining+ supervised fine-tuning
Indeed as we define above, especially for the recent foundation models, the pre-training phase on large datasets follows unsupervised fashion, i.e. for general knowledge, then the fine-tuning phase tailors the model into specific applications. However before this stage, reducing the horizon of the scale, we are already using supervised pretraining and fine-tuning for typical deep learning tasks.
Pros and Cons
If pre-training is the great journey across the general knowledge, then fine-tuning is the delicate craft of specialization. We promised fun and challenges for each approach, now it’s time to check them out.
Pros of Pre-Training
- Full control over the model: Pre-training gives you complete flexibility in designing the architecture and learning objectives, enabling the model to suit your specific needs.
- Task-generalized learning: Since pre-trained models learn from vast, diverse datasets, they develop a rich and generalized understanding that can transfer across multiple tasks.
- Potential for state-of-the-art performance: Starting from scratch allows for new innovations in the model structure, potentially pushing the boundaries of what AI can achieve.
Cons of Pre-Training
- High resource cost: Pre-training is computationally intensive, often requiring large-scale infrastructure (like cloud servers or specialized hardware) and extensive time.
- Vast amounts of data required: For meaningful pre-training, you need enormous datasets, which can be difficult or expensive to acquire for niche applications.
- Extended development time: Pre-training models from scratch can take weeks or even months, significantly slowing down the time to market.
The pros and cons of fine-tuning are straightforward by flipping the coin. In addition to that, we also mention a few nuances.
Pros of Fine-Tuning
- Straightforward:lower resource requirements, less strict data requirement.
- Faster to market: This becomes critical if you are running an AI business and would like to take one step faster than the competitors
- Flexible with target domains and tasks.Your AI applications and business may vary with time, or simply require a refinement of functionality. Fine-tuning makes that much easier.
Cons of Fine-Tuning
- Straightforward:less architectural flexibility, potential suboptimal performance.
- Model bias inheritance: Pre-trained models can sometimes carry biases from the datasets they were trained on. If your fine-tuned task is sensitive to fairness or requires unbiased predictions, this could be a concern. In general, the quality of fine-tuned models depends more or less on the pre-trained model.
When to Choose Fine-tuning
Fine-tuning is ideal when you want to leverage the power of large pre-trained models without the overhead of training from scratch. It’s particularly advantageous when your problem aligns with the general patterns already learned by the pre-trained model but requires some degree of customization to achieve optimal results. We raise several factors, with priority arranged in order:
- Limited resources: Either computational power or the dataset, if acquiring the resources is hard, fine-tuning a pre-trained model is more realistic.
- Sensitive timeline: Fine-tuning is mostly faster than pre-training, not just because of training from scratch. There are always risks that pre-training is sub-optimal and requires further refinement on the model architectures etc.
- Not necessarily the best model: If the performance requirements are not SOTA, but just a reliable, stable application of your foundation model, then fine-tuning is usually enough to achieve the goal. But it is considered much harder to beat all other models just by fine-tuning.
- Leverage existing frameworks: If your application fits well within existing frameworks (such as system compatibility), fine-tuning offers a simpler, more efficient solution.
Your Product’s Role
Inference acceleration refers to techniques that optimize the speed and efficiency of model predictions once the model is trained or fine-tuned. Whichever approach you choose, a faster inference time is always beneficial, both in the development stage and on market. We mention one major factor that the impact of inference acceleration on fine-tuning is more immediate.
- A Matter of Priority: During pre-training, the model’s complexity and computational demands are very high, and the primary concern is optimizing the learning process. The fine-tuning process, on the other hand, will soon move forward to the evaluation or deployment phase, when inference acceleration saves significant resources.
Inference Process of LLM
LLMs, particularly decoder-only models, use auto-regressive method to generate output sequences. This method generates tokens one at a time, where each step in the sequence requires the model to process the entire token history—both the input tokens and previously generated tokens. As the sequence length increases, the computational time required for each new token grows rapidly, making the process less efficient.
Inference Acceleration Methods
- Data-level acceleration: improve the efficiency via optimizing the input prompts (i.e., input compression) or better organizing the output content (i.e., output organization). This category of methods typically does not change the original model.
- Model-level acceleration: design an efficient model structure or compressing the pre-trained models in the inference process to improve its efficiency. This category of methods (1) often requires costly pre-training or a smaller amount of fine-tuning cost to retain or recover the model ability, and (2) is typically lossy in the model performance.
- System-level acceleration: optimize the inference engine or the serving system. This category of methods (1) does not involve costly model training, and (2) is typically lossless in model performance. The challenge is it also requires more efforts on the system design.
An Example of System-level Acceleration: WhaleFlux
WhaleFlux falls into the system-level acceleration, with a focus on GPU scheduling optimization. It is an open-source service for LLM deployment, monitoring, injection, and auto-scaling. Here are the major technical features and values:
- Automatic configuration recommendation.
- Real-time performance monitor.
- Stable, efficient and scalable. Increase resource utilization by over 50%and enhance comprehensive GPU memory utilization from 40% to 90%.
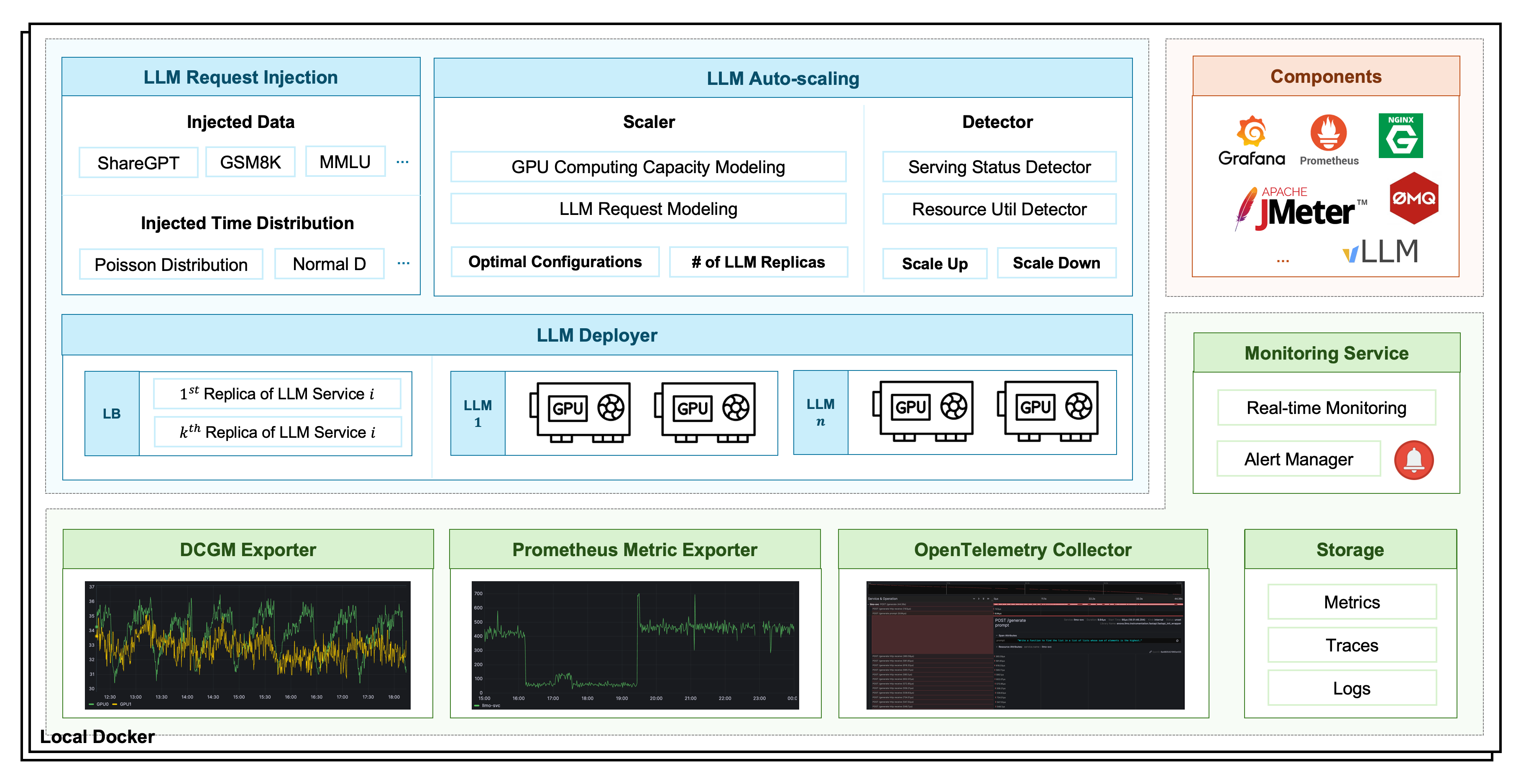
The figure above shows the components and functionalities of WhaleFlux. For more details we refer to the Github repo and the official website of WhaleFlux.
Conclusion
Choosing between fine-tuning and pre-training, by the end of day, depends on your specific project’s needs, resources, and goals. Fine-tuning is the go-to option for most businesses and developers who require fast, cost-effective solutions. On the other hand, pre-training is the preferred approach when developing novel AI applications that require deep customization, or when working with unique, domain-specific data. Though pre-training is more resource-intensive, it can lead to state-of-the-art performance and open the door to new innovations.
For practical concerns, most applications would favor inference acceleration techniques during pre-training and fine-tuning stages, especially for real-time predictions or deployment on edge devices. Data and model-level acceleration are more studied in the academic field, while system-level acceleration has more immediate effectiveness. We hope the content in this blog helps with your choice for your AI applications.